A very interesting paper has been published in IEEE Transactions on Intelligent Transportation Systems entitled “High Time-Resolution Queue Profile Estimation at Signalized Intersections Based on Extended Kalman Filtering” utilising the pNEUMA dataset.. The authors from Zhejiang University, Aalto University and University of Illinois at Urbana-Champaign, used both simulated and real-world data to evaluate the performance of their methodology.
Highlights
- A machine learning method is applied to construct a dynamic shockwave propagation model based on shockwave theory and historical data of Connected Vehicles
- A heuristic approach is proposed to measure the shockwave speed
- An urban queue estimator is designed to combine the dynamic shockwave propagation model and real-time shockwave information to deliver second-by-second queue profile estimates
Abstract
The dynamic spatiotemporal characteristics of queues at urban intersections are crucial to traffic operation tasks such as signal performance measure and signal optimization. This paper addresses the high time-resolution estimation of queue profile at urban signalized intersection using Extended Kalman Filtering (EKF) with data of connected vehicles (CVs). The main features of this work are as follows: (i) a machine learning method was applied to construct a dynamic shockwave propagation model based on shockwave theory and historical data of CVs; (ii) a heuristic approach was proposed to measure the shockwave speed for use in EKF; (iii) an urban queue estimator was designed to combine the dynamic shockwave propagation model and real-time shockwave information via EKF to deliver second- by-second queue profile estimates. The queue estimator does not require any priori information about vehicle arrival patterns and the market penetration rate (MPR) of CVs. The performance and robustness of the queue estimator were evaluated using both simulation and real-world CV data. The results show that the method can provide satisfactory queue estimation results at various MPR levels of CVs, with the estimation error of 2.5 vehicles at the MPR of 5%, and of 0.5 vehicle at the MPR of 40%.
You can read the paper here.
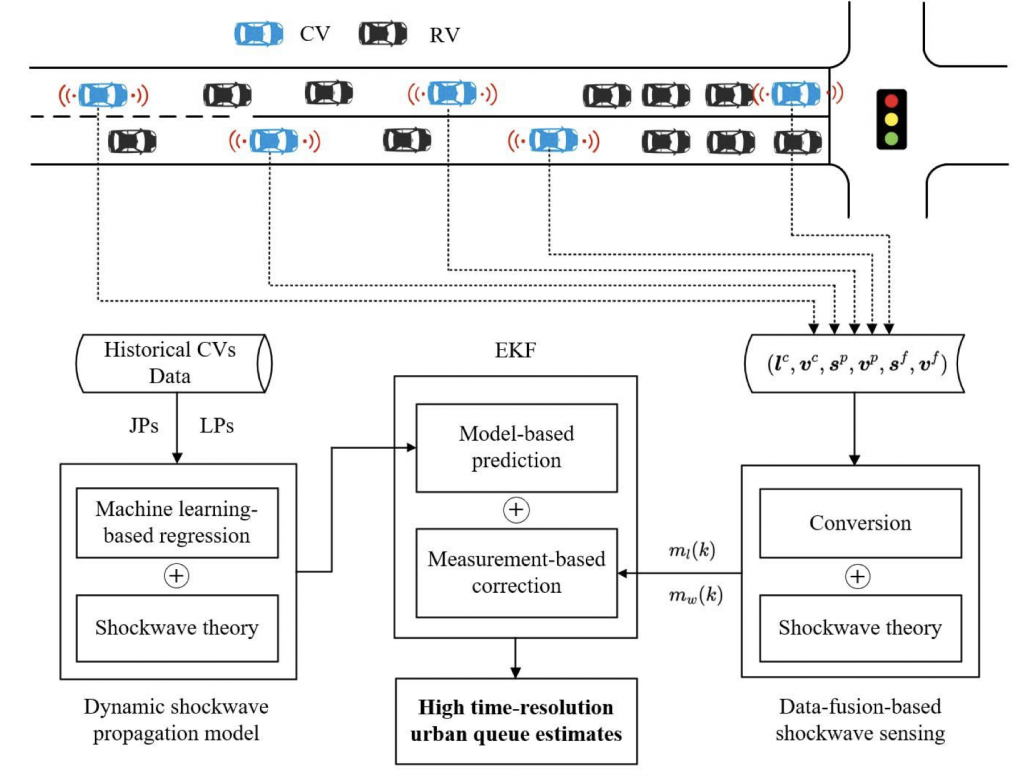